SMs, Cores and Warps
- A GPU has a number of Streaming Multiprocessors (SMs) which have a number of cores. Threads are scheduled in units of Warps
- Each SM has a number of resources
- E.g 400-500 series Nvidia GPUs have:
- 2 Instruction despatch units
- Each can start, on each clock cycle, processing on any 2 banks at a time. The 3 banks of 16 cores mean that 2 sequential instructions from one thread warp can be executing simultaneously if are not dependent on each other
- 3 banks of 16 cores (48 cores)
- 1 bank of 16 load store units
- for calculating source and destination addresses
- 1 bank of 4 special functional units
- hardware support for calculating sin, cos, reciprocals and square roots
- 1 bank of 4 texture units
- Global Memory and constant memory is the only GPU memory that you can copy into.
- constant memory can only be edited by the host
<< ./deviceQuery
>> ./DeviceQuery/Debug/deviceQuery Starting...
Device 0: "GeForce RTX 2070"
CUDA Driver Version / Runtime Version 10.1 / 10.1
CUDA Capability Major/Minor version number: 7.5
Total amount of global memory: 7982 MBytes (8370061312 bytes)
(36) Multiprocessors, ( 64) CUDA Cores/MP: 2304 CUDA Cores
GPU Max Clock rate: 1710 MHz (1.71 GHz)
Memory Clock rate: 7001 Mhz
Memory Bus Width: 256-bit
L2 Cache Size: 4194304 bytes
Maximum Texture Dimension Size (x,y,z) 1D=(131072), 2D=(131072, 65536), 3D=(16384, 16384, 16384)
Maximum Layered 1D Texture Size, (num) layers 1D=(32768), 2048 layers
Maximum Layered 2D Texture Size, (num) layers 2D=(32768, 32768), 2048 layers
Total amount of constant memory: 65536 bytes
Total amount of shared memory per block: 49152 bytes
Total number of registers available per block: 65536
Warp size: 32
Maximum number of threads per multiprocessor: 1024
Maximum number of threads per block: 1024
Max dimension size of a thread block (x,y,z): (1024, 1024, 64)
Max dimension size of a grid size (x,y,z): (2147483647, 65535, 65535)
Maximum memory pitch: 2147483647 bytes
Texture alignment: 512 bytes
Concurrent copy and kernel execution: Yes with 3 copy engine(s)
Run time limit on kernels: No
Integrated GPU sharing Host Memory: No
Support host page-locked memory mapping: Yes
Alignment requirement for Surfaces: Yes
Device has ECC support: Disabled
Device supports Unified Addressing (UVA): Yes
Device supports Compute Preemption: Yes
Supports Cooperative Kernel Launch: Yes
Supports MultiDevice Co-op Kernel Launch: Yes
Device PCI Domain ID / Bus ID / location ID: 0 / 11 / 0
Note:
- 1 core = 1 processing unit and can execute 1 thread
- 1 Warp = 32 cores
Key details from printout above:
- 36 SMs
- 64 cores/SM (=2304 CUDA cores total)
- Maximum of 1024 threads/ SM
- Maximum of 1024 threads/block
- Maximum GPU clock rate of 1.71 GHz
When a kernel is invoked with a configuration of Grid, Blocks and Threads
- Each block is assigned to a SM (streaming multi-processor) based on availability
- Max 1024 threads/block ⟹ 2 blocks can be in flight on each SM
- This can be increased by having less threads allocated to each block
- When a block is assigned to a SM, the warps of the block are distributed statically among the warp schedulers
- With a maximum of 1024 threads/SM (32 warps) each warp scheduler gets at most 8 warps (due to each SM having 4 warp schedulers << Check)
- Maximum number of blocks/SM is ??
- Each scheduler can issue to to 2 independent instruction from the same warp each cycle
- Warps execute in lock-step
- so synchronisation within a warp is mostly automatic but (data variables should be marked
volatile
)
- In practice, it is much more complex
- We need to be able to synchronise threads in a block
- We also need to be able to synchronise threads in a warp
- Cannot synchronise across different blocks
- Barrier synchronisation:
__syncthreads()
- Must Never have
__syncthreads()
in a branch of a conditional that some threads in the block will not execute
- else a deadlock will occur.
Warp Execution and divergence
A whole warp is handled by a single controller. Consider what happens if some threads A in a warp take one branch, 1, of an if
statement and others B take the other branch, 2.
- All threads in the warp must execute the same instructions
- The first branch, 1 : here all threads execute branch 1 instructions. However, the threads in group B are disabled (analogous to de-clutching a car)
- Then execute branch 2: again, all threads execute the instructions but branch A threads are disabled.
- This is called divergence
- The whole warp execute the same branch ⟹ NO Divergence
Loops where different threads in the warp execute different numbers of iterations also form divergences. I.e. the threads that conceptually execute the lowest number of iterations actually execute the same as the rest of the threads, conceptually the same as the threads executing the most iterations.
Divergence and Reduction
Now we will look at the consequences of divergence for the process of reducing a set of numbers to 1. E.g. ∑x=11024x
- Sequentially we would solve this by iterating through a vector, accumulating the sum in a variable
- In parallel, one approach would be to use a binary tournament:
- Round 1:
- thread 0 executes
A[0] += A[1]
- thread 2 executes
A[2] += A[3]
- ⋯
- thread 2i, where 0<2i<1024, executes
A[2*i] += A[2*i+1]
- Round 2:
- thread 4i, where 0<4i<1024, executes
A[4*i] += A[4*i+2]
- ⋯
- Round n
- thread 2ni executes
A[pow(2,n)*i] += A[pow(2,n)*i+pow(2,n-1)]
Diagrammatically, this would look like:
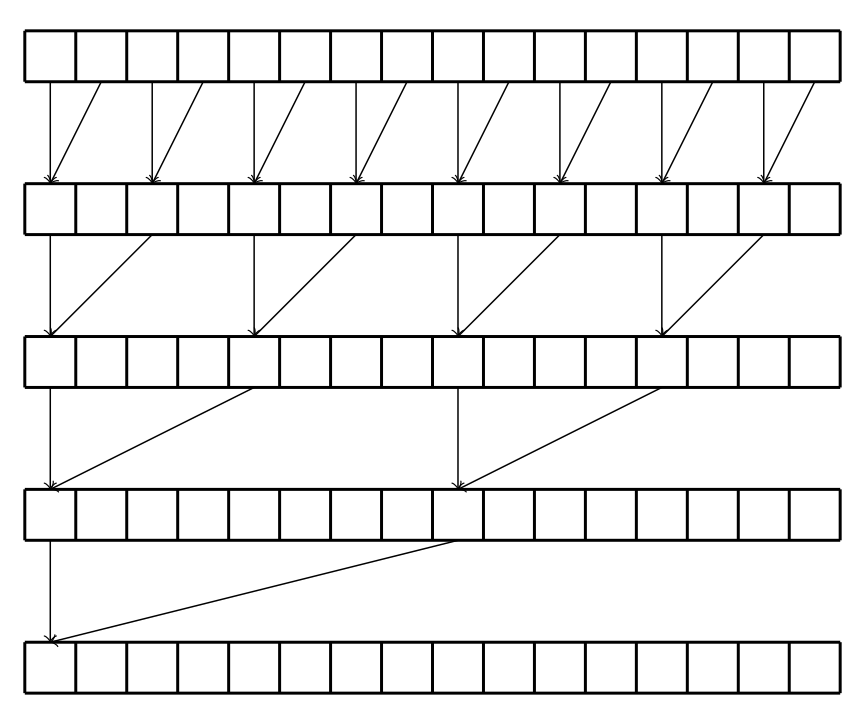
This could be implemented as follows:
float partialSum[];
...
uint t = threadIdx.x;
for (uint stride = 1 ; stride < blockDim.x; stride *= 2){
__syncthreads()
if (t % (2 * stride) == 0){
partialSum[t] += partialSum[t+stride];
}
}
- Assume
blockDim.x = 1024
__syncthreads()
is required to make sure all threads have completed the previous stage before continuing
- In first pass, all warps are active but only half the threads are doing useful work. This only gets worse on future iterations
Diagrammatically an alternative, more efficient, method could look like:
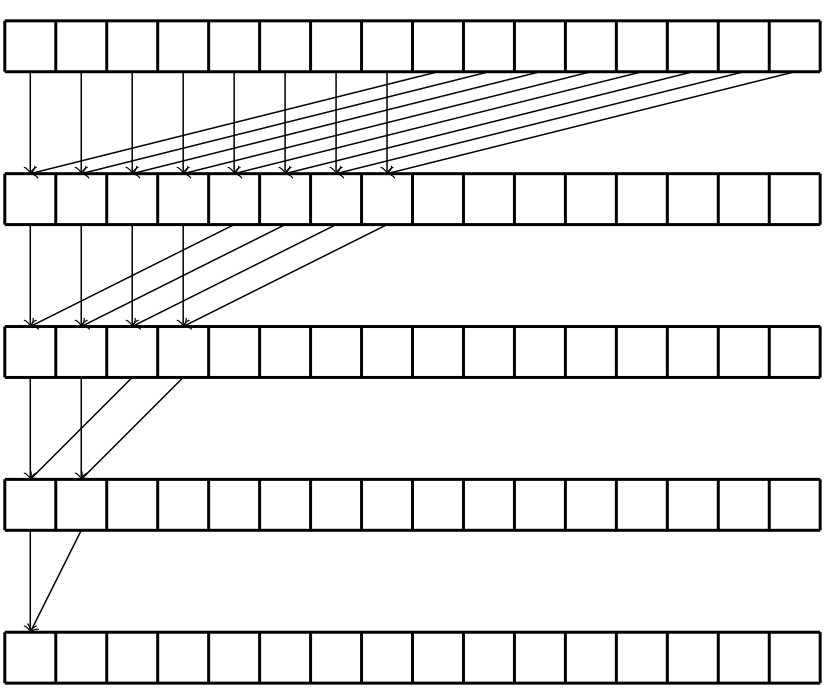
with the corresponding code looking like:
float partialSum[];
...
uint t = threadIdx.x;
for (uint stride = blockDim.x/2 ; stride > 0 ; stride >> 1){
__syncthreads();
if (t<stride){
partialSum[t] += partialSum[t+stride];
}
}
- Again, assuming that
blockDim.x = 1024
- Here however, threads 0-511 (warps 0-15) execute the true branch with threads 512-1023 executing the false branch, this leads to no divergence, therefore 1 pass each iteration
- Continues 1 pass each iteration until there are less than 32 threads executing
- For simple operations such as vectorAdd the compute to global memory access ratio or CGMA is 1/3, i.e. 1 flop per 3 memory accesses
- Assume the global memory access bandwidth is in the range of 200GB/s and the processor can execute around 1500 GFLOPS then the memory bandwidth is limiting us in the following way:
- 3 read/writes = 12 bytes
- 12 bytes memory access at 200GB/s for each flop = 200/12 GFLOPS = 17 GFLOPS
- Therefore, although the hardware is capable fo 1500GFLOPS, our global memory limits us to just 17 GFLOPS
Applying this to our parallel reduction:
- Each reduction reads 2 words from global memory and wrtites one word back to global memory per working thread
- If instead the first read copied from global → shared memory, the remainder of the operation would be much faster
- In general, many such operations exist whereby, a large problem can be broken into small tiles, each of which fits into shared memory, the tiles can be worked on and combined at the very end for a much faster overall calculation.